Skills Matter
Longform content marketing | Content strategy | UX Copywriting
Keeping a lid on hate
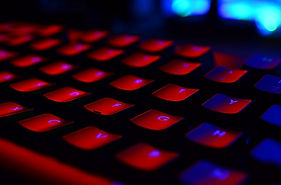
Beyond data concerns, expansion goals, or VR technology, there is one major concern on Mark Zuckerberg's mind and that is content moderation. As reported in a Motherboard article last August, Facebook’s founder held a number of high-profile dinners with social media academics last year to talk about various issues facing the tech giant, but none more so than how to keep a lid on hate.
The article’s authors hint at an interesting dichotomy between Zuckerberg’s stated goal, for Facebook to be “one global community”, and reality: Facebook’s 2.32 billion monthly active users are comprised of a near countless number of individual communities, languages, norms, dialects, religions and more. Moderating this online ecosystem according to one ‘ideal’ is Herculean at best and Pyrrhic at worst.
One famous instance of this almost impossible task was a training document given to content moderators. They were presented with three groups — ‘female drivers’, ‘black children’, and ‘white men’ — and asked which group was protected from hate speech. The correct answer, at that time, was ‘white men’, simply because both words (‘white’ and ‘men’) were both protected terms, according to race and sex, and acted in unison to form a protected category. The other two options did not meet this criteria. This was quickly addressed once pointed out, but distills the problematic and ceaseless challenge of moderation online.
Why do our tech giants care so much about content moderation? Because they don’t want to lose users. Tech companies like Facebook and Twitter are somewhat protected by law (section 230 of the Telecommunications Act) from legal action for the content posted by its users. This is different across the globe. In Europe, for instance, hosting providers are not responsible for the content they host if they are not made aware of its illegality or don’t act promptly to remove it once made aware. As a business, however, moderation is front-of-mind if they want to keep their product (users) growing and their stakeholders happy.
Outside of Facebook (and its subsidiaries) and Twitter, there are organisations that work with these companies that work to a higher ideal, and offer a much greater insight into the challenges and nuances of content moderation than any strenuous interview with the CEO of Twitter can.
Co-founder of Hatebase, Timothy Quinn, offered me a much deeper insight into content moderation than anything I could find in terms of what Mark Zuckerberg or Jack Dorsey have to say.
I could explain what Hatebase does, but there is nothing better than seeing a website with clear copy as its main heading, explaining exactly what it is:
Hatebase predominantly uses this repository of hate speech language to help NGOs tackle the threat of genocide and physical violence towards minorities within communities, as well as to help companies in their own content moderation.
Most companies don’t have the internal resources or linguistic expertise to automate the detection of discriminatory user content Timothy saw how impactful the language of one particular radio program had been in fuelling real violence during the Rwandan Genocide, and sees a direct correlation between that technology and today’s social media platforms in their potential for fuelling physical violence.
“Our approach is to help companies extend their existing capabilities by using Hatebase data and analysis to automate some portion of their moderation flow,” he explains.
And they need help. Facebook has at least 7,500 human moderators working across the globe, placing upon them the immense pressure to make snap judgements about the approximate 1.4 million daily rule-breaking posts that take place on their platform.
“Image, decision. Image, decision. Image, decision.”
That’s how an American content moderator who worked for Facebook described his job when talking to Radiolab for a podcast they did on content moderation. He had to process an image every 3–4 seconds for 8 hours a day and decide whether each one was appropriate for the internet or not. This only related to images and doesn’t even cover the realm of language.
For Timothy at Hatebase, the scale of the problem for companies is gargantuan and is exactly why their needs to be resources that can explore and detail the complexities of language to help keep both online and real communities safe.
“Most companies don’t have the internal resources or linguistic expertise to automate the detection of discriminatory user content, but there’s a huge penalty for not doing this.
“Allowing hate speech to remain online pollutes their ecosystems for legitimate users and risks incurring legal liabilities because of hate speech regulations, which can be particularly severe in countries like Germany which have recently cracked down on companies that operate within their borders.
“Our vision is that assessing hate speech should be as quick and seamless as, say, validating an email address or geolocating a user’s IP address to set language preferences.
“At the same time, as defenders of free speech, we’re cautious about casting the net too widely and unintentionally inhibiting dissent. For example, our data isn’t designed to flag a user who writes “build the wall” even though this expression is more likely to denote xenophobia than a post containing the expression “build a door.” We do, however, use these sorts of shorthand terms and memes to inform our assessment of the context of a post if we see them in conjunction with a term which we know to be hate speech.”
This idea of context is key to the incremental honing of automatic moderation practices, especially when it comes to the training and inference of Machine Learning processes.
“A large percentage of the hate speech in our database is contextual in nature,” Timothy continues to explain to me.
“In other words, while it may be, or appear to be, benign in some contexts, it can have a hateful intent in other contexts. A good example of this is when a term is repurposed from one ethnicity and used against another ethnicity, like “aboki,” — a Hausa term for “friend” — which is sometimes used by other ethnicities in Nigeria to refer to Hausa people as uneducated. Similarly, a benign term can be taken from general usage and repurposed within an ethnic context, like Louis Farrakhan’s use of the word “termite” to refer to Jews.
“Hateful context becomes much more difficult to assess when people use words, memes, or imagery that, while arguably not themselves hate speech, are still informed by discriminatory intent. One way that we use this sort of language is through incorporating ‘helper’ language into our analysis. These are words and phrases that tell our automation that there’s an increased likelihood that a given piece of content contains hate speech.
“For instance, if Hatebase parsed a user post that referred to termites, it wouldn’t automatically know if the term was intended to refer to Jews or to insects. But if there are other ‘helper’ terms in the same post (e.g. “totenkopf,” the Nazi death’s head symbol), Hatebase will probably report an increased likelihood that the post is hateful in context.”
This essentially returns us to the complexities that confused rookie moderators at Facebook when asked to differentiate between ‘white men’ and ‘black children’ as those requiring protection from hate speech, and hints at the importance of maintaining a human element in a process that monitors human speech patterns. To think that Machine Learning could one day effectively monitor and police language calls into question the role of both technology and language as human tools.
Yet, the sheer scale of potentially inflammatory, defamatory, abusive or threatening content produced each day on the internet is too big an ask for a human workforce, which Radiolab revealed to be working in conditions that often lead to PTSD. For Timothy Quinn, automatic processes can play a role in reducing the load from the worst cases of hate speech.
“There will always be a role for both automated and human moderation. One of our primary goals with Hatebase has been to help organizations strengthen their automated moderation so as to reduce the burden placed on human moderators, because manual moderation is an expensive, stressful, Sisyphean investment of time and money for most organizations.
“Our approach to solving the moderation problem has been to continually add nuance to the data that we stream out, and to incrementally tweak the performance of HateBrain, our natural language parsing engine.
“For example, certain terms (everyone can think of one or two) are unambiguously hate speech in any context. There’s just no benign usage of those terms. Other terms are unambiguously hate speech in certain countries, so we’re alert for those as well. Certain terms are more likely to be hate speech when accompanied by other very specific words (particularly if those ‘helper’ words are inflammatory or xenophobic in nature), if the terms are obfuscated in some fashion, or if they’re accompanied by a specific emoji. Our platform looks for all of those attributes when making an automated assessment of hate speech context.
“Using this data, moderators can enhance their systems to help automatically identify the worst of the worst and then triage the remainder to human moderators depending on how likely it is that a given user post contains hate speech.”
Maintaining such vast databases of contextual information and criteria, this calls into question our relationship with data, privacy, and whether the seemingly fundamental idea of freedom of speech holds up against the weight of hate threatening to bubble up and upend the pot.
The current furore over content censorship shows just how much technology has kicked the rock over to reveal the complexities of our relationship with language. It has also revealed that our traditional belief in the internet as a place that should be free from constraint can result in far worse consequences than hurt feelings, and could even be akin to turning our backs on the potential for genocide. What is clear is that we cannot turn solely to ourselves or solely to machines to police each other, but trust in the ability for both to self-regulate.
